AI-Powered Rules as Code: Experiments with Public Benefits Policy: Summary + Key Takeaways
This is the summary version of a report that documents four experiments exploring if AI can be used to expedite the translation of SNAP and Medicaid policies into software code for implementation in public benefits eligibility and enrollment systems under a Rules as Code approach.
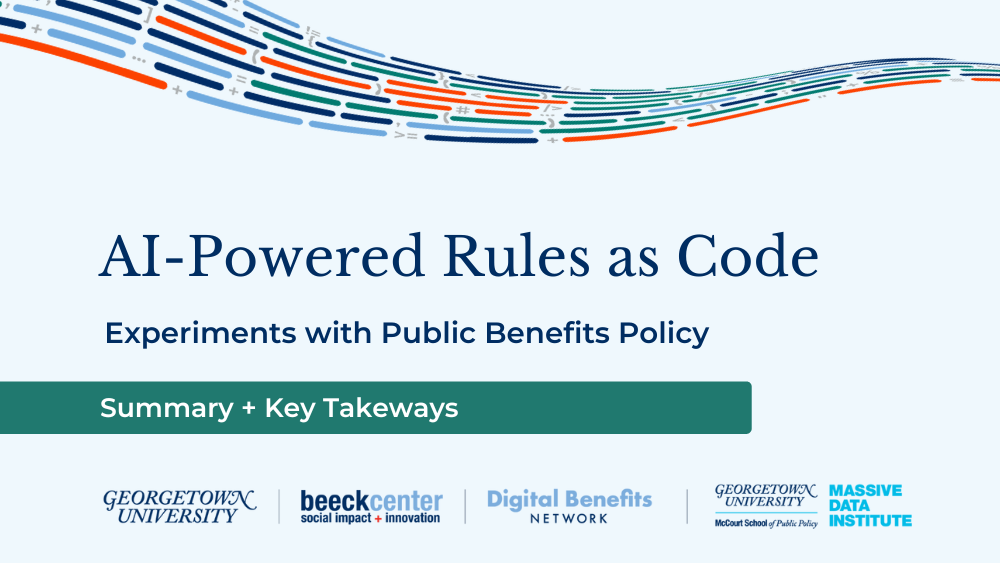
The rise of commercially-available large language models (LLMs) presents an opportunity to use artificial intelligence (AI) to expedite the translation of policies into software code for implementation in public benefits eligibility and enrollment systems under a Rules as Code approach.
Our team experimented with multiple LLMs and methodologies to determine how well the LLMs could translate Supplemental Nutrition Assistance Program (SNAP) and Medicaid policies across seven different states. We found that LLMs are capable of supporting the process of generating code from policy, but still require external knowledge and human oversight within an iterative process for any policies containing complex logic.Â
We present our full findings in this report.
We conducted initial experiments from June to September 2024 during the Policy2Code Prototyping Challenge. The challenge was hosted by the Digital Benefits Network and the Massive Data Institute, as part of the Rule as Code Community of Practice. Twelve teams from the U.S. and Canada participated in the resulting Policy2Code Demo Day. We finished running the experiments and completing the analysis from October 2024 to February 2025.Â
In the report and summary you will learn more about key takeaways including:
- LLMs can help support the Rules as Code pipeline. LLMs can extract programmable rules from policy by leveraging expert knowledge retrieved from policy documents and employing well-crafted templates.
- LLMs achieve better policy-to-code conversion when prompts are detailed and the policy logic is simple.
- State governments can make it easier for LLMs to use their policies by making them digitally accessible.
- Humans must be in the loop to review outputs from LLMs. Accuracy and equity considerations must outweigh efficiency in high-stakes benefits systems.
- Current web-based chatbots have mixed results, often risking incorrect information presented in a confident tone.
Share this Resource:
Content related to this resource:
AI-Powered Rules as Code: Experiments with Public Benefits Policy
This report documents four experiments exploring if AI can be used to expedite the translation of SNAP and Medicaid policies into software code for implementation in public benefits eligibility and enrollment systems under a Rules as Code approach.