Library
Discover the latest innovations, learn about promising practices, and find out what’s coming next with best-in-class resources from trusted sources.
Is there something missing from our library?
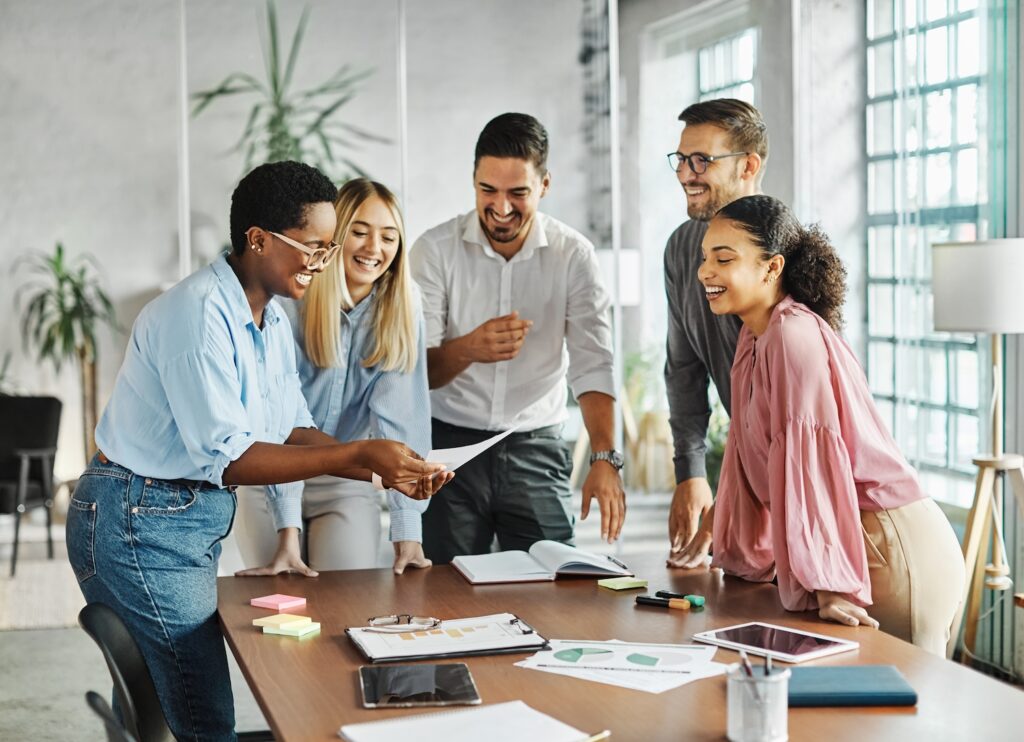
Search for the topic or resource you're looking for, or use the filters to narrow down results below.
-
Automation + AI Gender Shades: Intersectional Accuracy Disparities in Commercial Gender Classification
Recent studies demonstrate that machine learning algorithms can discriminate based on classes like race and gender. This academic study presents an approach to evaluate bias present in automated facial analysis algorithms and datasets.
-
Automation + AI The Privacy-Bias Tradeoff: Data Minimization and Racial Disparity Assessments in U.S. Government
An emerging concern in algorithmic fairness is the tension with privacy interests. Data minimization can restrict access to protected attributes, such as race and ethnicity, for bias assessment and mitigation. This paper examines how this “privacy-bias tradeoff” has become an important battleground for fairness assessments in the U.S. government and provides rich lessons for resolving these tradeoffs.
-
Automation + AI Algorithmic Impact Assessments and Accountability: The Co-construction of Impacts
Algorithmic impact assessments (AIAs) are an emergent form of accountability for organizations that build and deploy automated decision-support systems. This academic paper explores how to co-construct impacts that closely reflects harms, and emphasizes the need for input of various types of expertise and affected communities.
-
Automation + AI Surveillance, Discretion and Governance in Automated Welfare
This academic article develops a framework for evaluating whether and how automated decision-making welfare systems introduce new harms and burdens for claimants, focusing on an example case from Germany.
-
Automation + AI Popular Support for Balancing Equity and Efficiency in Resource Allocation
This article explores how online advertising algorithms bias between Spanish and English speakers for SNAP in California.
-
Automation + AI Defining and Demystifying Automated Decision Systems
This article suggests that a lack of clear, shared definitions makes it harder for the public and policymakers to evaluate and regulate technical systems that may have significant impacts on communities and individuals by shaping access to benefits, opportunities, and liberty. It presents and evaluates a definition for automated decision systems, developed through workshops with interdisciplinary scholars and practitioners.
-
Automation + AI Against Predictive Optimization: On the Legitimacy of Decision-Making Algorithms that Optimize Predictive Accuracy
This academic paper examines predictive optimization, a category of decision-making algorithms that use machine learning (ML) to predict future outcomes of interest about individuals. Through this examination, the authors explore how predictive optimization can raise concerns that make its use illegitimate and challenge claims about predictive optimization's accuracy, efficiency, and fairness.
-
Diversity, Equity + Inclusion Re-Envisioning Medicaid & CHIP as Anti-Racist Programs
This report puts forth an anti-racist reimagining of Medicaid and CHIP that actively reckons with the racist history of the Medicaid program and offers principles and recommendations that capitalize on the transformative potential of the programs. The principles center the voices and agency of program participants and prioritize direct community involvement at all stages of the policy process.
-
Digitizing Policy + Rules as Code Exploring Rules Communication: Moving Beyond Static Documents to Standardized Code for U.S. Public Benefits Programs
This brief analyzes the current state of federal and state government communication around benefits eligibility rules and policy and how these documents are being tracked and adapted into code by external organizations. This work includes comparisons between coded examples of policy and potential options for standardizing code based on established and emerging data standards, tools, and frameworks.
-
Human-Centered Design Administrative Burden Scale
The Better Government Lab at the McCourt School of Public Policy at Georgetown University has developed a new scale for measuring the experience of burden when accessing public benefits. They offer both a three-item scale and a single-item scale, which can be utilized for any public benefit program. The shorter scales provide a less burdensome way to measure by requiring less information from users.
-
Procurement Serving the Citizens—Not the Bureaucracy: A Strategic Vision for City Procurement
Report proposing that cities reimagine procurement as a public service, which can unlock a world of ideas for change and improvement.
-
Human-Centered Design Why Governments Should Prioritize UX for Everyone
Through our research understanding the government digital service field and what workers in this field need, we want to help strengthen those existing roles and establish more pathways for promotion and career support, as well as help other teams recognize the value of these skills and create new roles.