Library
Discover the latest innovations, learn about promising practices, and find out what’s coming next with best-in-class resources from trusted sources.
Is there something missing from our library?
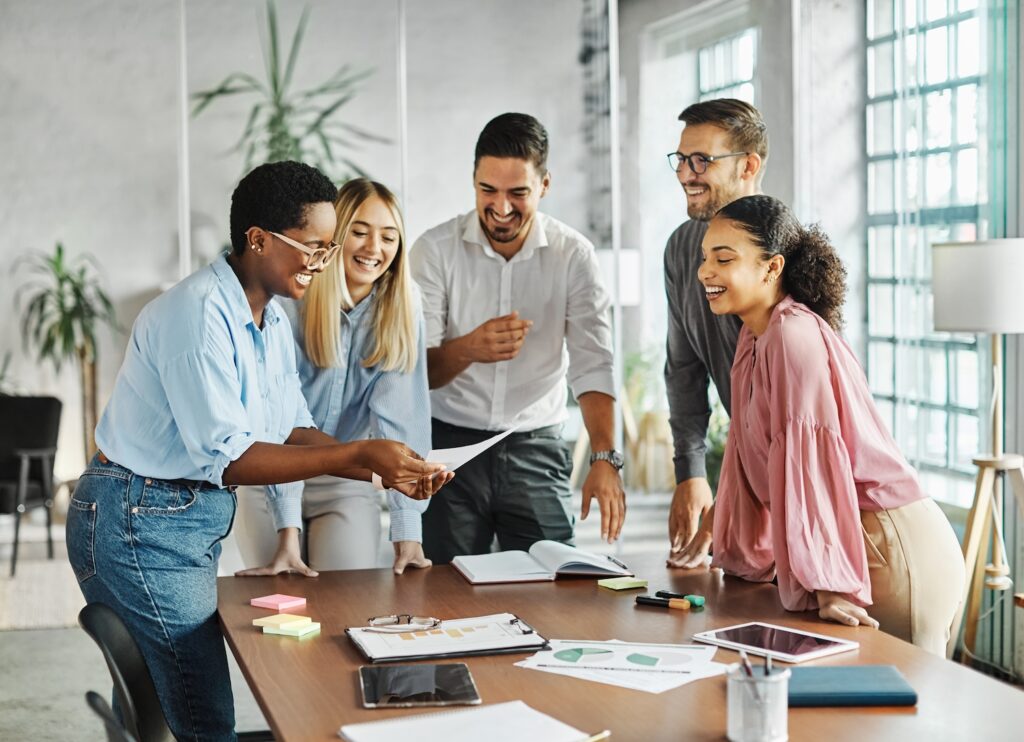
Search for the topic or resource you're looking for, or use the filters to narrow down results below.
-
Digital.gov User Testing and Research News and Events Feed
A collection of updates, news, and events on user testing and research from Digital.gov.
-
Digital.gov Research Resources
Resources from Digital.gov on research
-
18F Methods Collection
A collection of tools to bring human-centered design into your project.
-
Why Governments Should Prioritize UX for Everyone
Through our research understanding the government digital service field and what workers in this field need, we want to help strengthen those existing roles and establish more pathways for promotion and career support, as well as help other teams recognize the value of these skills and create new roles.
-
Usability Starter Kit
Here are some tools and templates to help you create better user experiences.
-
Tips for capturing the best data from user interviews
In this blog post, we’ve detailed some of the steps we take to help capture the best data possible when conducting interviews. This post is intended as a guide for people who need to conduct user interviews and for people simply curious about how we work.
-
Design Resource Tools
Use these activities, tools, and how-tos as a starting point — we hope you’ll hack them for whatever challenge you’re working on.
-
Barriers to Government’s Adoption of User-centered Design — And How To Address Them
In this article we’ll provide an overview of four of the most common barriers heard, and how federal agencies might go about addressing them.
-
Civic Service Design Tools & Tactics
Website with various articles regarding civic service design tools and tactics, case studies, and other articles.
-
7 Ways to Ignite User-Centered Design at Your Agency
Some tips for success when introducing user-centered design (UCD) to your organization.
-
City of St. Paul User Experience Participation Legislation
Policy from the City of Saint Paul to provide compensation to those participating in user experience research and usability testing.
-
How User Research Can Help
Blog discussing how user research can help improve your agency’s benefits implementation, service delivery workflows, and procurement.